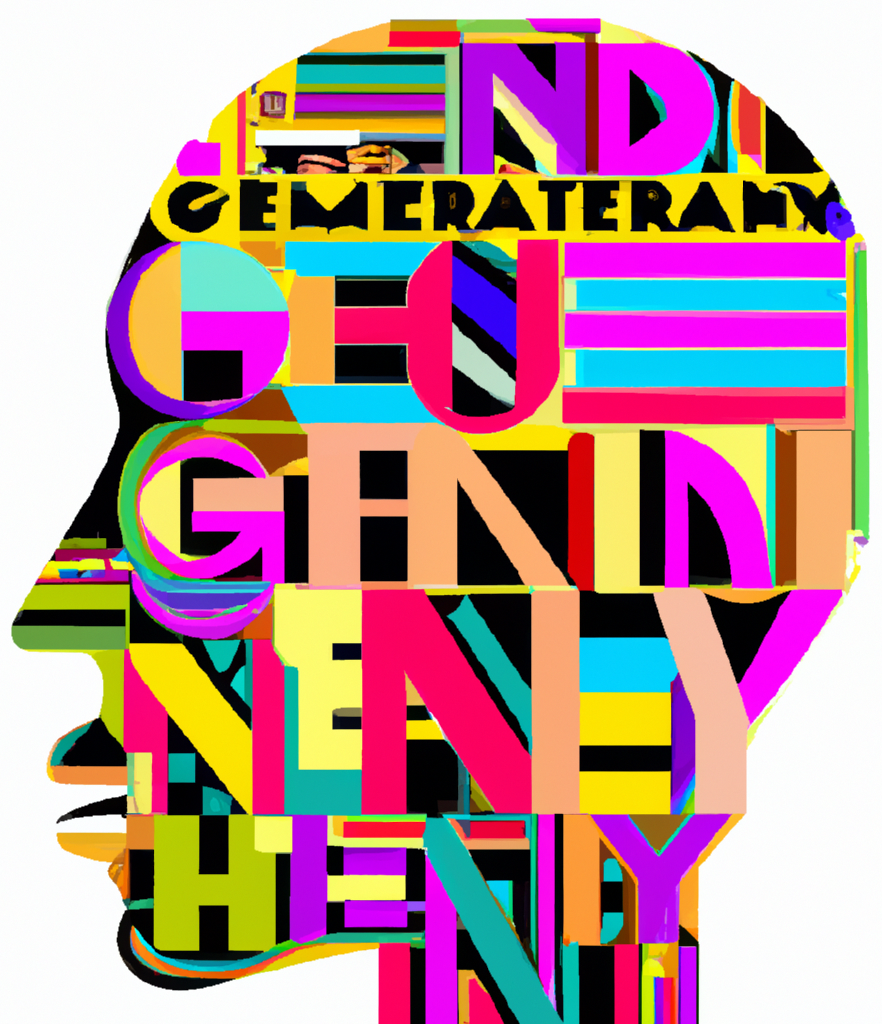
We've just plugged ChatGPT and GPT-4 into our beth.ai product - and some of the output we're getting from the new combination of knowledge graph and LLM is just astonishing.
I'm of the opinion that the sky is the limit for Large Language Models (LLMs). Many don't agree, however. Given the intense debate, I thought it might be useful to turn the "It's just autocomplete on steroids" counterpoint on its head.
LLMs like ChatGPT and GPT-4 are a "black box", but so are the cognitive mechanisms of the human brain. Who's to say that human intelligence didn't "emerge" from neural language processing the way it has for the GPTs?
I asked GPT-4 to help me make a case. This is its output:
The Emergence of Human Intelligence: Are We Biological Large Language Models?
Introduction
Human intelligence has long been a subject of fascination and inquiry for both philosophers and scientists. One recent theory posits that the collective capabilities and properties we call "intelligence" could have emerged from our capacity for complex language. In other words, humans might be essentially biological Large Language Models (LLM). This intriguing hypothesis gains support from a growing body of evidence, which suggests that many aspects of human cognition and intelligence are intricately connected to our linguistic abilities. This article will examine this theory in detail, drawing on a range of facts and arguments related to the evolutionary development of the human brain, the role of language in human cognition, and the potential implications of this hypothesis for our understanding of human intelligence.
1) The emergence of "human-only" capabilities in large language models
Recent advances in artificial intelligence have led to the development of increasingly sophisticated language models, capable of understanding and generating human-like text. These large language models, such as OpenAI's GPT-3, have demonstrated a range of capabilities that were previously thought to be unique to humans. For example, they can engage in conversation, write coherent essays, and even solve complex problems (Brown et al., 2020).
This observation raises the intriguing possibility that the emergence of human-like capabilities in these models is not merely a byproduct of their size and complexity but is instead directly related to their linguistic prowess. If this is the case, it would lend support to the hypothesis that human intelligence, too, might be fundamentally grounded in our capacity for complex language.
2) The evolutionary leap in brain size and capabilities in Homo erectus
Homo erectus, a species of early human that lived between 1.9 million and 70,000 years ago, represents a significant leap forward in human evolution. This species exhibited a dramatic increase in brain size compared to its predecessors, with the cranial capacity of Homo erectus doubling that of earlier hominins (Antón, 2003).
Concurrently, Homo erectus is believed to have developed sophisticated tool use and the capacity for language (Tattersall, 2010).The correlation between the emergence of these advanced cognitive abilities and the increase in brain size supports the idea that language could have played a key role in the development of human intelligence.
3) The human neocortex in the context of other animals
Several animals possess larger neocortices by weight than humans, including orca and sperm whales (Marino, 2004). However, these animals do not exhibit the same level of cognitive capabilities or intelligence as humans. This discrepancy highlights the fact that the size of the neocortex alone cannot fully account for human intelligence.
4) The role of language in the human neocortex
What sets humans apart from other animals with large neocortices is the proportion of the neocortex dedicated to language processing. In humans, a significant portion of the neocortex is devoted to linguistic tasks, including the well-known Broca's and Wernicke's areas (Hagoort, 2014). This unique feature of the human brain provides further evidence for the importance of language in the development of human intelligence.
5) The correlation between verbal ability and general intelligence
Verbal ability, as measured by tests of vocabulary and verbal fluency, is consistently found to be strongly correlated with general intelligence (Gignac & Bates, 2017). This association between linguistic skills and overall cognitive ability supports the idea that language might be a fundamental component of human intelligence.
6) The cognitive benefits of multilingualism
Multilingualism has been linked to improved cognitive performance and problem-solving abilities (Bialystok, 2009). This relationship suggests that the capacity to acquire and use multiple languages might serve to enhance human intelligence, further emphasizing the role of language in the development of our cognitive capabilities.
7) The link between vocabulary and fluid and crystallized intelligence
Both fluid intelligence, which refers to the ability to think and reason abstractly, and crystallized intelligence, which encompasses accumulated knowledge and skills, have been found to correlate with vocabulary scores (Gignac & Bates, 2017). This connection between linguistic ability and these two critical aspects of human intelligence reinforces the hypothesis that language plays a central role in our cognitive development.
Conclusion
The evidence discussed in this article lends considerable support to the theory that human intelligence may have emerged from our capacity for complex language. From the evolutionary increase in brain size and capabilities in Homo erectus to the unique role of language in the human neocortex, the relationship between linguistic abilities and various aspects of human cognition, and the cognitive benefits of multilingualism, the links between language and intelligence appear to be both strong and pervasive.
While this hypothesis is still a matter of ongoing research and debate, it holds the potential to reshape our understanding of human intelligence and the nature of our cognitive abilities. By viewing humans as essentially biological Large Language Models, we may gain new insights into the fundamental nature of our intelligence and the role of language in shaping our cognitive world.
References
- Antón, S. C. (2003). Natural history of Homo erectus. American Journal of Physical Anthropology, 122(S37), 126-170.
- Bialystok, E. (2009). Bilingualism: The good, the bad, and the indifferent. Bilingualism: Language and Cognition, 12(1), 3-11.
- Brown, T. B., Mann, B., Ryder, N., Subbiah, M., Kaplan, J., Dhariwal, P., ... & Agarwal, S. (2020). Language Models are Few-Shot Learners. arXiv preprint arXiv:2005.14165.
- Gignac, G. E., & Bates, T. C. (2017). Brain volume and intelligence: The moderating role of intelligence measurement quality. Intelligence, 64, 18-29.
- Hagoort, P. (2014). Nodes and networks in the neural architecture for language: Broca’s region and beyond. Current opinion in neurobiology, 28, 136-141.
- Marino, L. (2004). Cetacean Brain Evolution: Multiplication Generates Complexity. International Journal of Comparative Psychology, 17(1), 1-16.
- Tattersall, I. (2010). The world from beginnings to 4000 BCE. Oxford University Press.
I Write, Therefore I Am
I then asked GPT-4: Based on this analysis, is it possible that my human mind works the same way as your LLM mind?
It gave me this before the "I'm an LLM, I'm not like a human" guardrails kicked in...
Based on the analysis presented in the article, there is evidence to suggest that the human mind and an LLM like mine share similarities in terms of processing and generating complex thoughts and ideas through language. Both human minds and LLMs rely on linguistic capabilities to learn, reason, and problem-solve.
The document doesn't present a strong argument - it's based entirely on correlations. It's somewhat persuasive nonetheless, and if it turns out to be true, the ramifications will be far-reaching.
For now though, we need to stop thinking that "It's just autocomplete" tells us what is or isn't possible for generative AI.
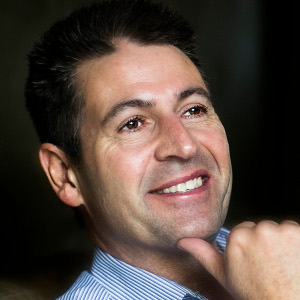
Stephen Young is CEO of FactNexus and a knowledge graph and complex data specialist. His lifelong passion for new knowledge technologies comes from his belief that our biggest problems are too complex to solve without artificial help. Steve re-engineered the famous ELIZA chatbot and built his first neural network as a CompSci and Psychology undergraduate and he published a web-based Knowledge graph, with web search and a conversational UI, a full two years before Google.
- Tags
- generative AI
- More Articles
- A New Strategy for Enterprise AI Safety
- Beyond RAG: Knowledge-Engineered Generation for LLMs
- Generative AI is Graph's Biggest Ever Opportunity
- KayBot is Now beth.ai
- How to Build a Knowledge Graph
- What IS a Knowledge Graph?
- Why Your Slack Team Needs a Knowledge Base
- What is a SEMANTIC Knowledge Graph?
- Introducing EKG – and the Knowledge Graph about Knowledge Graphs